Current Research Projects
1. How does environmental heterogeneity influence the genomic footprint of adaptation?
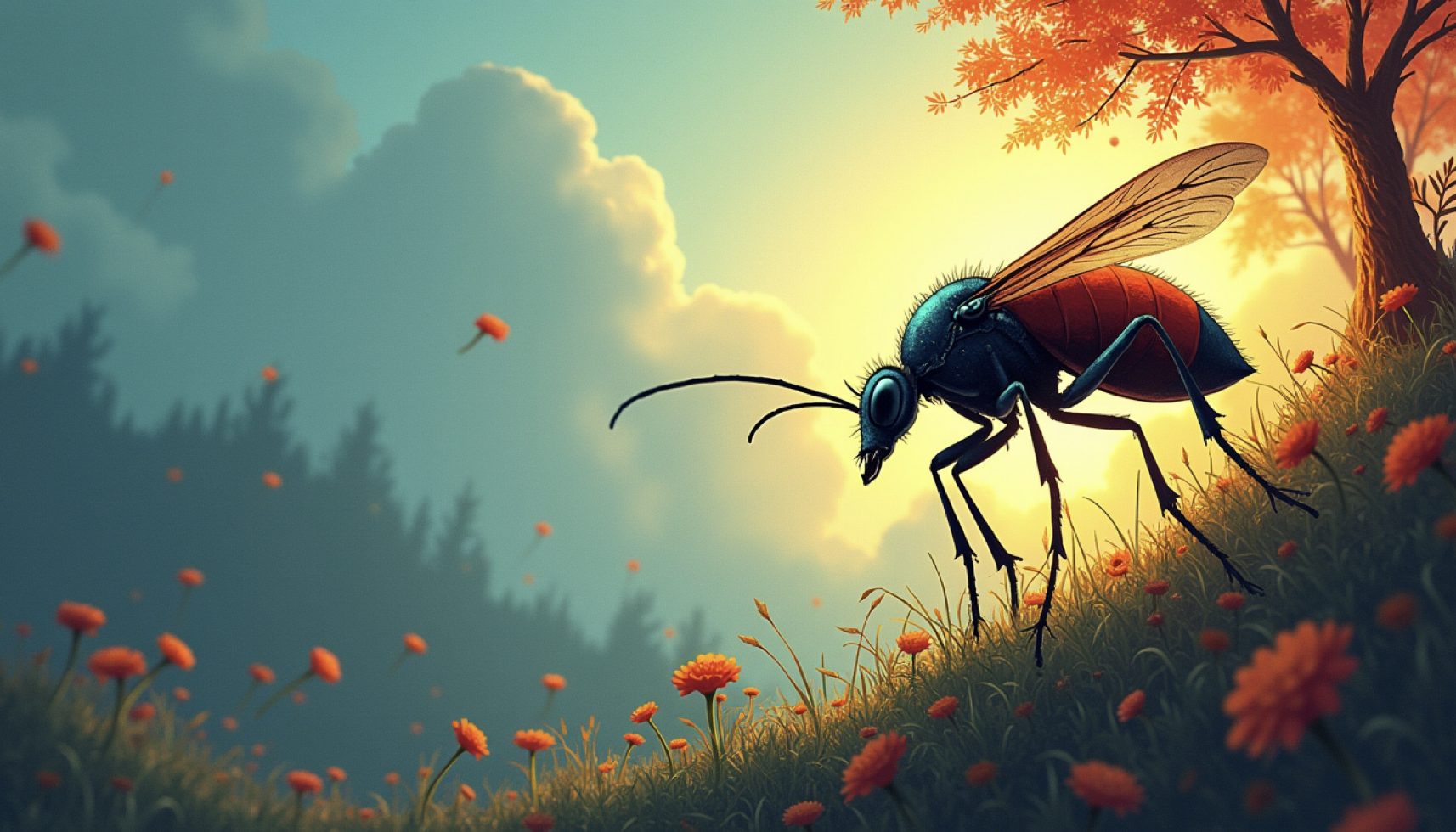
Understanding how organisms adapt to rapidly changing environments is crucial, especially in the context of global climate change. Adaptation often involves evolutionary trade-offs, where adjusting to one challenge, such as insecticides, may affect other traits like heat tolerance. My research investigates how environmental heterogeneity influences the spread of adaptive mutations, considering these pleiotropic effects. In this context, classic population genetic models only provide limited insights as they assume random mating and spatial homogeneity. To address this, I employ individual-based simulations and statistical frameworks to explore how environmental heterogeneity shapes the genomic footprint of insecticide resistance and affects adaptation dynamics across time and space.
2. How does purifying selection shape transposable element invasions?
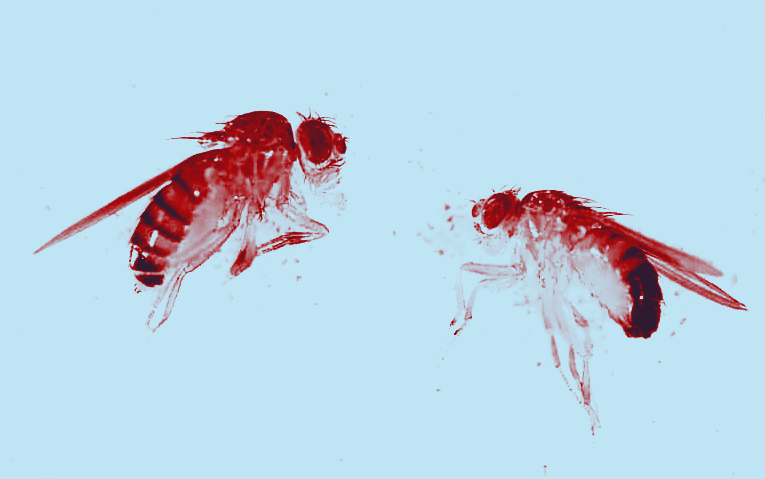
I study transposable elements (TEs), DNA sequences that can replicate within their host genomes. While the underrepresentation of TEs in coding regions suggests that purifying selection acts against new insertions, little is known about the balance between mutational pressure from TEs and the selection opposing them. My research investigates the distribution of fitness effects of new TE insertions in Drosophila simulans, using experimental evolution combined with statistical emulation. Focusing on the P-element, a well-known TE in Drosophila species, I show that most P-element insertions are deleterious and subject to strong purifying selection. This work highlights the power of combining experimental evolution, individual-based modeling, and machine learning to uncover the complex dynamics of TE invasions.
Click here to access the preprint
3. How does population structure influence host-pathogen dynamics in mosquito-transmitted diseases?
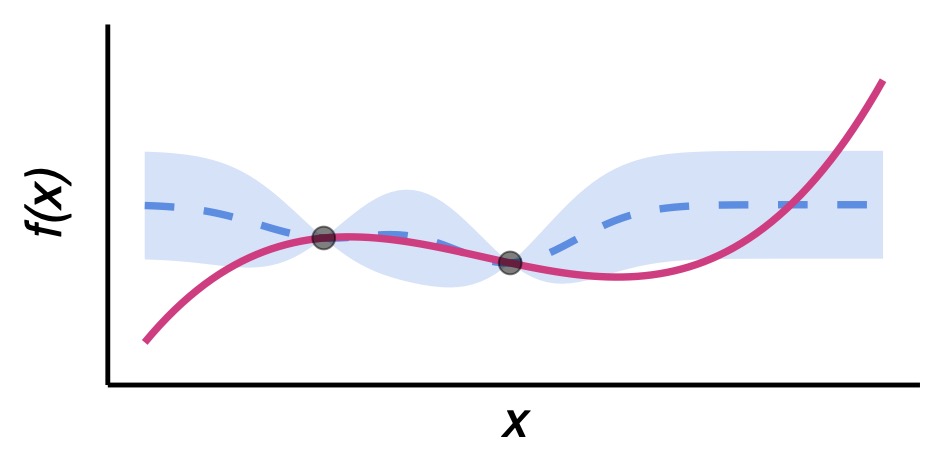
Individual-based models (IBMs) with a high level of biological realism can offer important insights into disease dynamics but are often too computationally expensive for systematic analysis. In this project, I explore Gaussian Process (GP) emulation to overcome this challenge. I developed an IBM loosely inspired by dengue, incorporating key drivers of dengue epidemics such as social structure, human mobility, and seasonality. GP surrogate models trained to predict outbreak probability, peak incidence, and epidemic duration enable rapid model analysis across the original model’s eight-dimensional parameter space. This paves the way for a comprehensive variance-based sensitivity analysis, which shows that average human mobility as a key driver of simulated epidemic outbreaks. Using a dataset of 1,000+ dengue outbreaks in Colombia for GP calibration, I pinpointed municipalities with consistently high infectivity levels. This work demonstrates how statistical emulation can make complex IBMs tractable and facilitate (empirical) data analysis.
Click here to access the preprint